How Predictive Analytics Enhances AI Customer Service
In the ever-evolving world of customer service, businesses are under constant pressure to provide faster, more personalized, and efficient solutions. While AI has already made significant strides in streamlining operations, integrating predictive analytics into AI customer service takes it to the next level. Predictive analytics leverages historical data and advanced algorithms to forecast customer behavior and needs, allowing companies to proactively address issues before they arise.
In this blog, we’ll explore how predictive analytics enhances AI customer service, from improving response times to offering personalized experiences that increase customer satisfaction and loyalty.
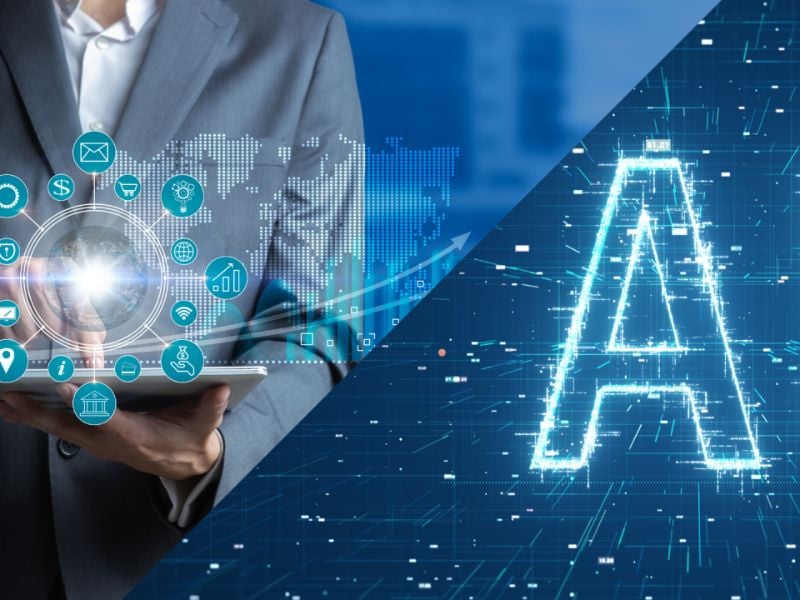
What is Predictive Analytics in AI Customer Service?
Predictive analytics involves using statistical algorithms, machine learning models, and historical data to identify patterns and make predictions about future events. In the context of AI customer service, predictive analytics helps anticipate customer needs, identify potential issues, and suggest solutions before a customer even reaches out.
By processing vast amounts of data from previous interactions, purchasing behavior, and even social media activity, AI systems can predict a customer’s next move and offer timely support that is highly relevant to their needs.
How Predictive Analytics Enhances AI Customer Service
1. Proactive Issue Resolution
One of the most powerful ways predictive analytics enhances AI-driven customer service is by allowing businesses to resolve issues before they escalate. AI systems can predict when a customer is likely to encounter a problem or when an issue will require attention.
Example: If an AI system detects that a customer’s order is delayed or out of stock, it can automatically notify the customer, provide an estimated delivery date, or offer alternative products.
Impact: By proactively addressing potential issues, businesses reduce frustration and prevent negative experiences, improving customer satisfaction.
2. Personalized Customer Interactions
Predictive analytics helps AI systems offer personalized experiences by analyzing past behaviors and preferences. This data enables AI-powered tools to make smarter recommendations, tailor responses, and provide more relevant assistance.
Example: An AI chatbot in an e-commerce setting can suggest products based on previous purchases or browsing history, increasing the likelihood of a sale.
Impact: Personalized interactions create a more engaging and relevant experience for customers, fostering loyalty and trust. When customers feel that their needs are anticipated and understood, their overall satisfaction improves.
3. Improved Response Times
Predictive analytics allows AI to anticipate customer queries, enabling faster response times. AI systems can prioritize issues that need immediate attention and triage routine inquiries to be addressed by automated solutions.
Example: By analyzing patterns in customer inquiries, an AI system can recognize peak times and adjust staffing or automation levels accordingly, ensuring prompt responses during high-demand periods.
Impact: Reduced wait times and quicker resolutions improve the customer experience, making support feel more efficient and responsive.
4. Reducing Customer Effort
Predictive analytics can minimize the effort required from customers to get the help they need. AI can automatically fill in customer details or offer pre-emptive solutions based on previous interactions, reducing the number of steps needed to solve a problem.
Example: If a customer reaches out about a refund, AI can predict the likely issue based on their previous purchase and streamline the process, offering a solution before the customer even has to explain the situation.
Impact: This leads to less frustration, as customers don’t have to repeat themselves or navigate complicated systems to get help, ultimately improving satisfaction.
5. Anticipating Customer Needs
AI systems equipped with predictive analytics don’t just respond to customer requests—they can predict what a customer might need next. By analyzing data from previous interactions, AI can forecast potential future needs, ensuring that customers receive the right help at the right time.
Example: In a telecom setting, AI might predict that a customer is likely to require an upgrade based on usage patterns and proactively offer tailored options before the customer needs to inquire.
Impact: Anticipating needs improves the customer journey by reducing friction and ensuring that support feels tailored and timely.
6. Optimizing Self-Service Options
With predictive analytics, AI can guide customers toward the most efficient self-service options, empowering them to solve issues on their own without needing to engage a live agent. This can significantly reduce operational costs while improving the customer experience.
Example: If a customer is likely to need assistance with account management, AI can preemptively offer a step-by-step guide or direct them to an appropriate FAQ section.
Impact: Customers appreciate the ease of access to helpful resources, while businesses benefit from reduced workloads for customer service agents.
Benefits of Using Predictive Analytics in AI Customer Service
1. Increased Efficiency and Reduced Costs
By predicting customer issues and needs, AI systems can streamline support processes and reduce the workload on human agents. Predictive analytics helps automate routine tasks and ensures that resources are used where they are needed most.
Example: AI can predict spikes in customer inquiries and automatically scale customer service teams or deploy more virtual assistants to handle routine requests.
Impact: Reduced operational costs and improved resource allocation lead to more efficient service and lower overhead for businesses.
2. Enhanced Customer Satisfaction and Loyalty
Proactively addressing customer needs, personalizing interactions, and providing faster solutions all contribute to a more positive customer experience. The result is increased satisfaction and, over time, stronger customer loyalty.
Example: A customer who receives a personalized recommendation based on previous interactions may feel more valued, leading them to return for future purchases.
Impact: Happy customers are more likely to become repeat customers and recommend your business to others, driving long-term growth.
3. Better Decision Making
By analyzing customer data, predictive analytics gives businesses deeper insights into customer behavior, preferences, and pain points. These insights can inform business decisions, helping companies improve their products, services, and overall customer experience.
Example: If predictive analytics reveals that customers often ask for a particular feature or report a recurring issue, businesses can prioritize addressing that feedback in future product updates.
Impact: Data-driven decision-making improves product offerings and customer experiences, which can differentiate a brand in a competitive market.
Case Studies: Predictive Analytics in AI Customer Service
1. Netflix
Netflix uses predictive analytics to personalize movie and TV show recommendations based on viewing history, ratings, and user preferences. AI algorithms predict what a customer will enjoy, making the customer experience more personalized and efficient.
Impact: By anticipating customer preferences, Netflix can improve customer satisfaction and reduce churn, as users are more likely to stay engaged with content they enjoy.
2. Amazon
Amazon uses predictive analytics to forecast demand and recommend products. AI predicts which products customers are likely to buy based on browsing history and shopping behavior, helping Amazon enhance the customer journey and optimize inventory management.
Impact: This predictive capability increases sales, improves customer satisfaction with relevant recommendations, and ensures timely order fulfillment.
Challenges of Predictive Analytics in AI Customer Service
While predictive analytics offers numerous benefits, there are some challenges to consider:
1. Data Privacy and Security
Using customer data to predict needs raises concerns about data privacy and security. It’s essential for businesses to ensure that customer data is handled responsibly and in compliance with regulations like GDPR.
2. Accuracy of Predictions
The effectiveness of predictive analytics depends on the quality and accuracy of the data it analyzes. Inaccurate predictions can lead to frustrating customer experiences and diminish trust in the AI system.
3. Integration with Existing Systems
Integrating predictive analytics with legacy systems or existing customer service platforms can be complex and require significant investment in technology and training.
Conclusion
Predictive analytics is a game-changer in the world of AI customer service. By leveraging historical data, AI can anticipate customer needs, offer personalized interactions, and resolve issues before they become problems. This not only improves response times and reduces costs but also enhances the overall customer experience, driving satisfaction and loyalty.
As businesses continue to evolve, incorporating predictive analytics into their AI strategies will be crucial for staying ahead of customer expectations and fostering long-term relationships. Embracing this powerful tool can help businesses deliver smarter, faster, and more personalized service to their customers.
喜欢我的作品吗?别忘了给予支持与赞赏,让我知道在创作的路上有你陪伴,一起延续这份热忱!
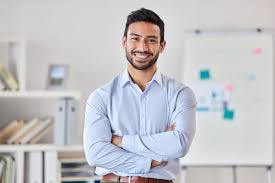
- 来自作者
- 相关推荐